Improving staging through machine learning based on multiparametric imaging: a collaborative platform
HOST INSTITUTION: Siemens Healthcare GmbH
Siemens Healthcare GmbH develops innovations that support better patient outcomes with greater efficiencies, giving providers the confidence they need to meet the clinical, operational and financial challenges of a changing healthcare landscape. As a global leader in medical imaging, laboratory diagnostics, and healthcare information technology, we have a keen understanding of the entire patient care continuum—from prevention and early detection to diagnosis and treatment. The company is headquartered in Erlangen, Germany. Siemens Healthcare GmbH operates as a subsidiary of Siemens AG.
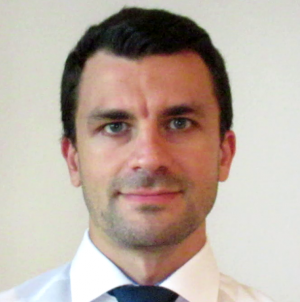
DESCRIPTION OF THE PROJECT (ESR12 - Nicolò Capobianco)
The ESR will develop a set of machine learning tools for collaboration across institutions that allow (1) systematic annotation of multimodality data (CT, MR, PET, SPECT); (2) integration of non-imaging data; (3) methods of standardizing data across patient datasets; and (4) sharing the pool of annotated data among research groups. In this phase the research goals will be focused on the methods of standardization, artefact identification and consistency checking between datasets and between sites, in order to enable broader and more reliable use. The second goal of the project is application of the framework to prostate cancer, as an illustration of the power of the platform.
Publications
Capobianco N, Sibille L, Chantadisai M, Gafita A, Langbein T, Platsch G, Solari EL, Shah V, Spottiswoode B, Eiber M, Weber WA, Navab N, Nekolla SG (2021) Whole-body uptake classification and prostate cancer staging in 68Ga-PSMA-11 PET/CT using dual-tracer learning. Eur J Nucl Med Mol Imaging. Jul 7. doi: https://doi.org/10.1007/s00259-021-05473-2. Epub ahead of print.
Capobianco N, Meignan MA, Cottereau AS, Vercellino L, Sibille L, Spottiswoode B, Zuehlsdorff S, Casasnovas O, Thieblemont C, and Buvat I (2020) Deep learning FDG uptake classification enables total metabolic tumor volume estimation in diffuse large B-cell lymphoma [published online ahead of print, 2020 Jun 12]. J Nucl Med. 2020; jnumed.120.242412. https://doi.org/10.2967/jnumed.120.242412
Nicolò wrote a summary of his publication that you can find here.
Presentation
EANM20: 'Whole-body lesion detection and prostate cancer staging in 68Ga-PSMA-11 PET/CT using deep learning'